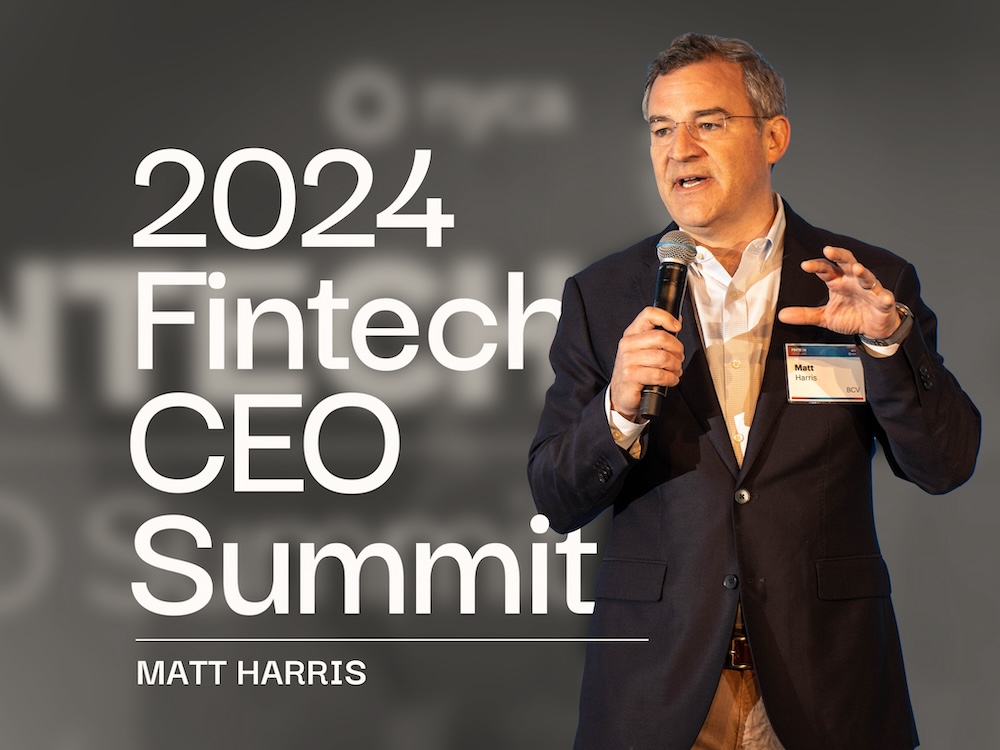
Only Up From Here: 2024’s State of Fintech and the Hero’s Journey
We’re at the scary part of “the hero’s journey” in fintech, with a bottom for funding and a BaaS crisis, but we’re about to enter a new, exciting stage.
The BCV fintech partnership shares our comprehensive perspective on how generative artificial intelligence is reshaping the financial services industry.
In this comprehensive piece, we’ll share what we’ve learned about generative artificial intelligence in financial services these last several months. You can use the following hyperlinked directory to jump around. Note that an * on a company’s name indicates it is a BCV portfolio company.
In January of this year, our partner Sarah wrote a piece considering the potential impact of the generative AI revolution on the financial services industry: “How Fintech Can Jump on the Generative AI Bandwagon.” At the time, the financial services ecosystem was eerily silent, even though ChatGPT had been released to the public a couple months earlier, and Twitter and articles all over the startup ecosystem were abuzz with grand prophecies of the profound impact generative artificial intelligence would have across the tech world.
She wrote:
As an investor who spends a lot of time in fintech, one thing that struck me about the coverage of generative AI has been how infrequently applications to financial services are discussed… Why is fintech not feeling the love?
Her piece was one of the early thought pieces on generative AI for fintech. In it, she identified the inherent incompatibility between the conservative financial services industry, which requires perfection for regulatory and business underwriting considerations, on the one hand, and the permissive nature of generative AI, on the other, which provides the “next best” token prediction and is by definition not precise or accurate. While this tension was true, she argued that there was still tremendous opportunity for generative AI to revolutionize the distribution, manufacturing and servicing of financial services. How? By not seeing generative AI as an end unto itself, but instead by “delivering generative AI as a component within the broader software or workflow process.” After we published we wondered: Would any of this actually come to fruition?
We have come a long way in the past four months! Since then, we’ve been fortunate enough to have a multitude of conversations with others searching for answers at this exciting time — including the heads of household-name financial services institutions, the teams leading AI efforts at established fintechs, and early stage founders creating something in a brand new space. Along the way, we’ve been able to refine our views through a series of presentations, debates and conferences that have taken us around the world. These conversations have been incredibly energizing for two main reasons: (1) It feels like history is being made, as the magnitude of the inevitable impact of generative AI on financial services is almost unfathomable, and (2) this field is moving quickly and at a depth that requires constant attention.
Within financial services organizations, AI is nothing new. In his 2022 letter to shareholders, JPMorgan Chase CEO Jamie Dimon famously wrote that the bank was spending “hundreds of millions of dollars” on AI and seeing identifiable returns on its tech bets (spending $12 billion on technology in 2021 alone). Of course AI hasn’t been reserved for only the largest organizations either: a 2022 report published by NVIDIA on the state of AI in financial services described AI use as “pervasive,” reporting that across all sectors of financial services — capital markets, investment banking, retail banking, and fintech — over 75% of companies utilize at least one of the core accelerated computing use cases of machine learning, deep learning and high-performance computing.
Already high, this number is rising: NVIDIA has also found that ninety-one percent of financial services companies around the world are currently leveraging AI investments to drive critical business — a leading indicator for expanded AI usage going forward.
If AI is nothing new for financial services organizations, why is generative AI getting so much attention? The “traditional AI” that financial services firms have effectively deployed for the last 40 years is very good at some tasks, but has its limits. Simply put, generative AI can do things that “traditional” AI cannot because it’s more flexible, both in terms of the data inputs required and the tasks performed.
As generative AI has exploded onto the scene, we’re seeing that financial services organizations are experimenting with generative AI while maintaining their investments in more traditional forms of AI. Generative AI cannot replace the role of traditional AI within financial services organizations because:
In this way, generative AI is neither an evolution nor a revolution. While evolution is the gradual development of something, especially from a simple to a complex form, revolution is the overthrow of the current system in favor of a new one. In both cases, the assumption is that it’s out with the old and in with the new. That’s not the case here. As we’re seeing play out today, we expect generative AI to coexist with and complement traditional forms of AI within financial services organizations for the foreseeable future.
Our team’s initial piece argued that there was the opportunity for generative AI to play a role in financial services organizations. Based on the conversations of the past few months, it’s time to make a far bolder argument:
Financial services organizations need to leverage generative AI to fulfill their potential for customers, employees and shareholders.
As sand can fill the open space in a jar of stones, generative AI can fill the gaps within financial services organizations left unfulfilled by traditional AI. These gaps today represent the unfulfilled potential of financial services organizations to offer better, higher quality, less expensive experiences for customers, more rewarding and fulfilling experiences for employees and better earnings for shareholders. It may be that traditional AI has not been directed toward these problems before, or that traditional AI has been insufficient, as in the case of customer service.
As explored in #1 above, financial services organizations have already invested heavily in technology, including traditional AI. In spite of this significant investment, however, there is still so much low-hanging fruit to create better experiences for customers and employees and to improve organizational profitability. Financial services is one of the industries with the lowest customer satisfaction scores and highest service costs. How can both be true?
How might generative AI change this low customer satisfaction and high service cost dynamic in financial services organizations today?
We’ve heard the phrase “platform shift” thrown out a lot in conversations around generative AI. “Platform” is just a fancy word for the model through which you’re interacting with a computer or other form of technology. The platform shifts we’ve seen over time include desktop to mobile and on-premise to cloud.
Generative AI represents a platform shift because it offers a fundamentally a new way to interact with technology. Because the underlying large language models are trained on an Internet-scale corpus of English text, we can interact with them using plain, everyday English. We’ve never been able to do this before! In the past, we’ve had to learn programming languages to interact with technology through code. And even during the Software 2.0 era, a lot of work went into feature engineering and hand-picking different deep learning architectures for different tasks.
Today, transformer-based models generalize amazingly well to a wide variety of tasks, making self-supervised learning far more feasible. The upshot for most users is that we can now interact with AI in our native tongue. (And back to #2, the language based nature of the generative AI platform is also why for the first time the AI is emotionally available to us!) For a more detailed discussion of the implications of this paradigm shift, check out Simon Taylor’s recent post.
However, we do not think that language replaces our interaction interface with all technology, especially in financial services where #, $ and % rule supreme. As mentioned in #1, we expect generative AI to co-exist with and complement traditional forms of AI within financial services organizations for the foreseeable future, like sand seeping into the gaps among the stones that have been left by traditional forms of AI and other technology.
Going back to the list of applications of “traditional” predictive AI within financial services organizations covered in #2, each of these could be enhanced with generative AI. The question and answer interaction style of the popularized generative AI chatbot can itself be embedded into other software, communication and AI experiences so that the generative AI is simply just a part of the fabric of the software. Tools like Microsoft 365 Copilot in Excel or Arkifi allow financial analysts to utilize a Q&A chatbot to output financial models and/or summarize key trends found in financial/numeric data. These analysts will still need to guide the AI to generate the most effective output, which will require their continued understanding of #, $ and %. As such, at least in the financial services context, generative AI is best suited as an embedded technology — a fundamental building block that will be part of (most) of the finance applications we experience going forward.
And, looking forward, we are excited to see teams innovate on the user interface beyond chatbots to distribute generative AI. The core innovation of generative AI is the next token prediction for large language models, and there are alternative methods to expose the predictions. A rose by any other name would smell as sweet!
Consider you’re hosting a birthday party and plan to serve birthday cake. What are your options?
It’s likely that what you choose to do depends on a few factors, such as your goal, your budget, the ingredients you already have at home, how much time you have available, when you’re thinking about this vs when the party is, any food sensitivities/allergies, and your skills. And it’s a certainty that not even the most ambitious, most able cooks are doing everything from scratch — we’re not going to be milking a cow and churning butter for this cake.
Similarly, there is a range of models available for a given use case of embedded generative AI in financial services. Over the course of dozens of conversations, we’ve seen three models emerge:
Relating this back to the factors that direct this choice of investment:
We expect that financial services organizations may simultaneously embed multiple use cases of generative AI throughout the organization. And, the way the use cases are implemented will probably span across the three models of Construct/Configure/Adopt. To extend the analogy: let’s say you’re also serving dinner at the party. You may choose to bake the cake from scratch, but order take-out pizza for dinner.
We’re often asked how financial services companies and fintechs are using generative AI today and wanted to share specific examples. We love answering this question because it shows both how far we’ve come and how far we have to go. H/T to our friends at a16z who have a great post on the five goals that generative AI is poised to fulfill for financial services organizations. In our field notes, we want to move beyond the theory and detail how financial services organizations are embracing generative AI in practice.
A few framing thoughts:
Based on what we’re seeing, what are our predictions for how financial services/fintech organizations will embed generative AI going forward?
Most of us are familiar with the “hype cycle” popularized by Gartner to describe the adoption of emerging technologies, shown below. Following a “technology trigger,” there’s a surge in interest and intent to integrate the technology, and the visibility of the technology rises to the “peak of inflated expectations,” or the top of the hype cycle. The other dimension shown on the graph below is utility; it’s still low through peak hype as companies are experimenting with and learning how to use the technology. The tendency of human nature toward over promising and under delivering results in the “trough of disillusionment” as companies realize the technology is actually not the cure-all as promised. Underneath, though, the real, careful work to integrate the technology is continuing to build, and the technology finds fit in its appropriate subset of use cases as its visibility rises once again through the “slope of enlightenment” and finally reaches the utility crescendo during the “plateau of productivity.”
Applying this to generative AI in the context of financial services: We don’t need to rehash the well-discussed “trigger event” created by technological progress from the transformer and diffusion models, or the very effective and accessible chat form factor from OpenAI that quickly exposed so many people to the technology, leading to a steep ramp in visibility. Rather than a new technology in the background, generative AI is AI in your face — we can see it, touch it and feel it. The network effects are wild — the novelty, combined with the early promise of utility, combined with the bewildering nature of it all — leads users to share their experiences with others… “Can you believe this?” “This is so helpful!” “I don’t know what to believe!” “Which app should I use? They all seem the same.” It’s a cycle that feeds itself to a frenzy at the top of the hype cycle, and feels like about where we are now.
In other words, we’re still early. Or, as our partner Christina poignantly put it in the Financial Times recently:
If ChatGPT is the iPhone, we’re seeing a lot of calculator apps. We’re looking for Uber.
However, it would also be a mistake to overlook that utility is building all the while. The value of inflated expectations is that it creates the activation energy that organizations need to overcome inertia — and this is especially important for financial services organizations that are inherently conservative.
Today, we’d be hard-pressed to find a single financial services or fintech company that has not discussed generative AI at their board meeting. It’s the topic that everyone wants to have on the agenda for industry conferences. Most companies have created a committee. In fact, even those organizations that are not particularly technologically-progressive have had to care because (1) generative AI is so easy for employees to use (via ChatGPT and other B2C apps) and (2) well-governed organizations have concerns about this use. These concerns include data privacy, regulation, enterprise data security, cultural issues around job security, hallucinations and impact on the brand, IP infringement, competition for talent, lack of recourse when there are not humans-in-the-loop, and cost and availability of GPUs. Oh, my! Many are already successfully wading through these concerns, and we’ve seen many examples of real business value in financial services and fintech organizations ‘in the wild’ (see #5 above).
That said, all of these examples are not created equally. Through our conversations, we’ve learned how to separate out the hype from the hope from what’s actually happening, and here’s how you can, too:
We’ve been struck by how frequently investors and operators seem to blend these three categories, seemingly taking at face value the generative AI announcements. Just like not all use cases are created equally, not all communications about generative AI integration are equally valid (or at least not yet).
For those organizations interested in proceeding from hype to hope, where do you start? We’ve seen that financial services companies and fintechs have had the most success integrating generative AI within very specific workflows or tools that they’re already using (vs. inventing wholly new use cases). More on how to begin in #9 below.
In summary, the Gartner hype cycle is a useful way to look at the advance of new technologies, including generative AI in financial services. The skeptics say it’s all hype. The believers say it’s changing the industry forever. Both are true. More than anything, we know it’s a time to be humble: In most cases when you hear about a generative AI use case in financial services or fintech, it’s just too early to tell. We recognize that most of the home-building has to happen below ground before we see the frame rise above ground.
Conversations about generative AI from a wide array of commentators routinely describe its powerful disruptive effects — e.g., BCG on March 7: “Generative AI has the potential to disrupt nearly every industry — promising both competitive advantage and creative destruction;” DataChazGPT, with close to 100,000 followers on Twitter on March 15: “The #AI disruption will happen faster than anything we’ve seen before;” Bill Gates on March 21: “Any new technology that’s so disruptive is bound to make people uneasy, and that’s certainly true with artificial intelligence;” and Goldman Sachs Asset Management on May 21: “We believe AI is a growth driver with a wide variety of applications and is on a path to disrupting entire industries as we currently know them.” But, these proclamations that generative AI is a disruptive technology in financial services are largely wrong.
If you’re only speaking to startups, it could seem that generative AI is a superpower that will enable David to topple Goliath. But, the startups are only part of the story, and we’re also speaking to the “incumbents” (here we mean both the large, established financial services companies, like Bloomberg, JPMorgan Chase and Charles Schwab, but also the established fintechs, like Stripe, AvidXchange* and Chime).
Typically, we use “disruption” to mean a radical change to the status quo, but the phrase “disruptive technologies” has a very specific meaning in business. In 1997, Clay Christensen published the “Innovator’s Dilemma,” defining the difference between “disruptive” and “sustaining” technologies. The Economist heralded the idea as “the most influential business idea of recent years.” It’s pretty clear when you return to Christensen’s original work that generative AI is primarily a sustaining technology. As Christensen defines:
Generative AI is by and large a sustaining technology because incumbents can effectively integrate it into existing products to improve the performance of these products in ways that are generally accepted by customers, e.g., by providing higher quality and more responsive customer service, by tailoring marketing messages more directly to individual users, by delivering a more complete and lower cost solution that requires less human intervention, etc.
As we’re seeing generative AI is defensibly used as a component of a product or software, rather than the product itself, the advantage generally lies with the incumbents that already have distribution and know the customer. So, not surprisingly, the vast majority of instances of generative AI being used in financial services and fintechs today, as shown in #5, are in existing companies, and not brand new startups.
Generative AI as a (mostly) sustaining technology is creating what some have described as a generative AI “arms race,” where well-funded incumbents are racing to establish the best partnerships, hire the best teams and develop the best new technology built on generative AI with their proprietary data.
Disruptive technologies, on the other hand, are difficult for established companies to invest in because 1) they create simpler and cheaper products, which generally have lower margins and don’t create more profitability, 2) are commercialized in emerging or non-core markets, and 3) most profitable customers don’t want to or can’t use the products. For this reason, the rational and well-managed incumbent will explicitly ignore disruptive technologies because it would be irrational to invest in these technologies!
As Christensen puts it: “Rational managers can rarely build a cogent case for entering small, poorly defined low-end markets that offer only lower profitability.”
However, incumbents will not universally benefit: There are instances in which we expect generative AI to be disruptive within the financial services industry, and therefore are interested in companies building in these spaces. We can map out the initial outline of where it is not in the best interest of the incumbents to offer a generative AI product, specifically:
These are collectively the Achilles heel of financial services incumbents in generative AI. We expect to see new companies founded with these attributes across financial services domains. We’d love to hear from you if you’re building a generative AI-native product that takes advantage of one or more of these vulnerabilities.
Before generative AI came onto the scene, some of the biggest trends in fintech were embedded finance and open banking. These haven’t gone away and, in fact, generative AI further accelerates these trends.
Embedded finance is all about delivery financial services in real time and digitally. It’s key to automate the manual tasks that are part of financial services distribution, and to help increase execution speed. Specifically, generative AI can help with fraud detection and risk management, personalized user engagement, customer support and knowledge retrieval. We’re particularly excited to see how generative AI can advance the embedded financial services promise in areas that have been more resistant to the embedded wave, such as insurance.
In the case of open banking, we believe generative AI will create more utility from open banking APIs. Startups (and incumbents) now have the opportunity to prompt better, leveraging real, specific, personal data. For example, Curl founder Mike Kelly recently demonstrated on LinkedIn how easy it is to connect generative AI and open banking when he created a “Bank-GPT” plug-in, which can tell customers their balance, find transactions, discuss budgeting and make payments. Consider this the beginning!
The worst place to start is nowhere; the best place to start is anywhere. While we need to be humble about sharing any “best practices” given how this entire field is developing as we speak, we have seen enough processes to share some practical advice. We’ve seen that how an organization begins will look different for every organization, but most simplify to a few steps:
The best way to learn what works is by engaging with others who are also on the journey. For example, at BCV we host an annual Fintech CEO Summit in addition to a Fintech Demo Day that brings together entrepreneurs (including several of our portfolio companies) building AI innovation, along with prospective partners within and across the financial services community. It should come as no surprise that AI has dominated these conversations over recent months. BCV also communes entrepreneurs sitting more squarely in the AI/ML arena; we recently launched BCV Labs, our technical community and incubator based in Palo Alto that’s focused on AI/ML innovation at both the application and infrastructure layers. Further, we’ve formed an AI/ML advisory board that meets regularly, and host events for our AI community in San Francisco and New York. (Please reach out to any of us if you’re interested in joining!)
Along the way, we’ve heard some common concerns from financial services organizations and fintechs — even among those that are currently in progress to embed generative AI. What follows is a summary of the most commonly cited concerns (albeit non-exhaustive), and some solutions (definitely non-exhaustive).
Let us leave you with one final idea: Doing nothing isn’t an option.
For anyone that wants to add more to this, or has ideas, or wants to compare notes, please get in touch! You can reach us at: shinkfuss@baincapital.com, cmelaskyriazi@baincapital.com, kzhang@baincapital.com, aco@baincapital.com, tdimitrova@baincapital.com, chowe@baincapital.com and mharris@baincapital.com.
We’re at the scary part of “the hero’s journey” in fintech, with a bottom for funding and a BaaS crisis, but we’re about to enter a new, exciting stage.
Relay is reinventing banking with more software and intelligence for every small business owner.
Generative AI can make companies more efficient, but customers have more to gain from it than they do — including in banking, commerce and medicine.