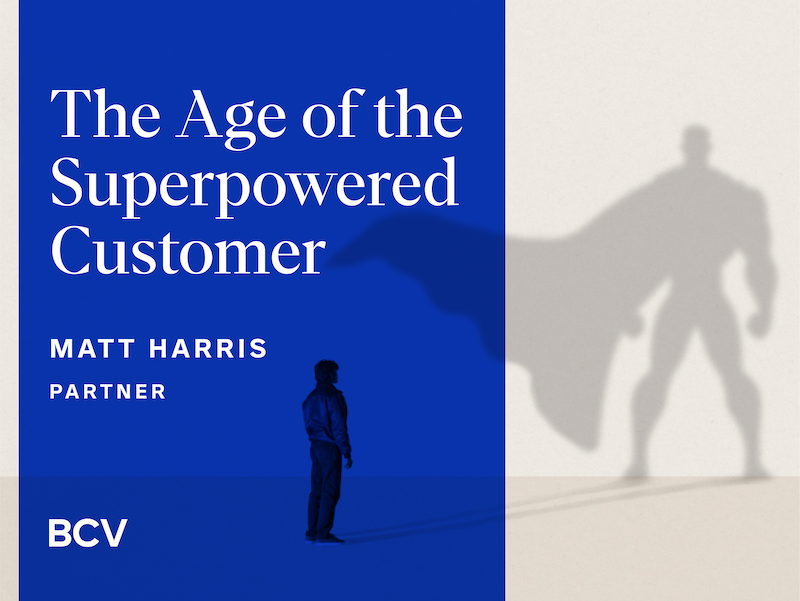
The Age of the Superpowered Customer
Generative AI can make companies more efficient, but customers have more to gain from it than they do — including in banking, commerce and medicine.
Generative AI can be a critical ingredient in the way financial services are delivered and make humans 10X more productive. In fact, there are many applications perfect for it.
There’s a reason financial services hasn’t been on the top of the list for generative AI companies: generative AI gives the 90% answer and financial services demands 100% accuracy. But it’s a mistake for generative AI to write off fintech, and vice versa. Generative AI can be a critical ingredient in the way financial services are delivered and make humans 10X more productive. In fact, there are many applications perfect for it.
In 2021, fintech was the darling of VC, accounting for $1 of every $5 of venture funding (CB Insights). Then fintech fell from grace in 2022. With inflation surging, the Fed began increasing interest rates in March, changing the fundamental economics under many companies, especially fintech companies whose performance is more directly tied to the cost of capital. Anxiety around the effects of the Durbin Act also rose and regulatory scrutiny increased, kicked off by meme stocks and the Robinhood drama. Putting it together, publicly traded fintech company valuations fell rapidly, some trading even below their cash reserves. According to CB Insights, global fintech funding was down 46% in 2022 compared to 2021. But, the narrative that fintech is dead is way too harsh as fintech still accounted for 18% of all venture dollars invested over the year (TechCrunch).
What has taken the place of fintech on the altar of venture investors? Generative AI. Generative AI as a concept has been around for awhile building off of technologies such as generative adversarial networks (GANs) developed in 2014, transformers in 2017, and Contrastive Language-Image Pre-training (CLIP) in 2021. The technology broke out in 2022 as models became much more performant and cost effective to train and serve, and their output improved considerably. Specifically, machine learning has long been able to outperform humans on perception and optimization tasks, but recent generative AI models have broken through the cognition barrier as well: how to make sense of data and information and understand them in context (Sifted). This has created what many have referred to as the ‘Cambrian Explosion of AI.’ Further, this technology was brought to all of us to play with: Open AI provided free tools for consumers (DALL-E, Chat GPT, etc.) in a short period of time and captured our attention and imagination. While the market is not large today at $8-10 billion, what has captivated investors is the anticipated growth in the market to more than $120B by 2030 (Grand View Research). If you’re interested to learn more about the state of the world of foundation models and AI, check out this great piece by my colleagues on the Infra team here at BCV.
As an investor who spends a lot of time in fintech, one thing that struck me about the coverage of generative AI has been how infrequently applications to financial services are discussed. For example, if you search for a market map of generative AI companies, you’ll see companies that focus on images and art, gaming, text for marketing and sales, and in the dev app space helping developers write code and also those focused on no-code. For example, check out this map from Dealroom or this one from CB Insights. Of course these categories somewhat cover financial services as the industry can still leverage these categories of AI as part of its sales and marketing copy processes or to streamline legal work, just as any company can. But, there seems to be little focus on the way that generative AI can transform the way that financial services products are distributed, underwritten, and serviced within the core financial services value chain. Why is fintech not feeling the love?
What I will say next will come as no surprise to any student of this industry: most financial services institutions will not be in the early adopters of generative AI writ large. Why? For the same reason as financial services institutions aren’t early adopters of most forms of technology: financial services institutions are conservative by design. The current zeitgeist is that generative AI is amazing and powerful, but it’s anything but accurate and precise, and since financial services has such high accuracy and precision requirements, it’s one of the last places that generative AI should be applied. Let’s explore this more.
As compared to most technology companies, the business model of financial services companies uniquely requires near perfection to avoid high cost outcomes. There is no room for error as financial services is a highly regulated industry where letting a bad apple through an otherwise perfect process can cost an institution their license to operate or ruin an insurer’s entire book of business. That’s game over. So, financial institutions are methodical, conscientious, preferring to (and sometimes required to by regulators) prolong time to value for the customer rather than letting a bad decision through. Important use cases that require 100% accuracy include underwriting, regulatory checks (AML, KYC, BSA/AML, OFAC screening), and accounting / financial reporting.
To illustrate the contrast, if a CRM SaaS company misprices a contract, the company may lose out on gross profit if the cost to serve is higher than expected or the willingness to pay is higher than expected. But, if a commercial insurance company misprices a cyber policy — or even worse underwrites a customer or asset that has risk that is just too high to price — the losses from this one policy could wipe out their entire book.
Back to generative AI, we’ve seen that it works well in processes that need to be “good enough” but not perfect, and financial services institutions won’t compromise on accuracy. So maybe it’s logical for generative AI to pass by financial services? It’s an idea whose time will come to the market, but just not now? Not so fast.
Within the emerging market of end apps built on top of foundation model APIs, the role of AI within the product varies quite a bit. Foundational LLM models are built on top of publicly available internet data, so are very good at tasks ‘out-of-the-box’ that require this generalized knowledge. More specialized outputs require a combination of specialized training data and specialized workflows or product wrappers around the AI. Put simply, all of these apps share the same core components:
How these apps vary is the relative thickness of the value provided by (2) and (3), where one end of the spectrum — we’ll call GaaS, or generative-AI-as-a-service— has a thin layer of (2) and (3) whereas the other end of the spectrum — we’ll call GaaC, or generative-AI-as-a-component — has a thick layer of (2) and/or (3).
For example on the GaaS side, Jasper uses GPT-3 to write blog posts and create original content for marketing and sales purposes in B2B and B2C contexts. The key value proposition is that the use of generative AI makes the copy automatic, and — while not 100% accurate — it is usually better anyway than copy today. As an example on the GaaC side of the spectrum, Notion recently launched Notion AI, a feature that embeds GPT-3 as a “digital assistant” into the notetaking and project collaboration workflows that Notion offers. Notion is making generative AI a component of their offering that improves the quality of their product.
Many of the first wave of generative AI apps companies that are seeing a lot of hype today are closer to the GaaS side of the spectrum. These companies are common in the first wave because they’re easier to create, relying most significantly on the foundational models. In contrast, it takes more development time to thicken the layer of model fine-tuning and/or wrap-around workflow for product delivery. As GaaS companies continue to mature, we expect they will build more and more intellectual property around their core to differentiate, proving out the ability to effectively use their own data for their specific purpose. The best among them will successfully evolve into GaaC companies (and thrive). In other words, as this market continues to mature, we’re likely to see more and more GaaC companies. The more the generative AI is an ingredient within a software product that solves a hard problem for an end market, the harder it is for the next smart, finely-tuned model to displace the company.
Turning back to financial services, for the foreseeable future, we expect that generative AI apps that successfully sell to financial services companies will be on the GaaC side of the spectrum, delivering AI as a component within the broader software or workflow process. There are two reasons for this. First, financial services data is not part of the publicly available internet datasets that have trained the foundational LLM models; these datasets are proprietary and private. So, apps leveraging the foundational models must fine-tune the models to make them relevant to the financial services use cases. Second, companies in this market will not buy generative AI to do a job because it’s not 100% accurate. Financial services companies will buy software (or services) to do a job, and the best software companies will leverage the best tools to make that job faster, cheaper, and better, including generative AI. In many cases, humans will still need to be in the loop, but the humans will be better informed, more efficient, and produce fewer errors with the assistance of generative AI. We argue that humans verifying vs creating is a huge value add.
And, it turns out, generative AI may be just what the financial services market needs to continue evolving.
My partner, Matt, has written about the evolution of fintech from discrete to embedded, broadly defined as non-financial services companies offering financial products and services. For example, our portfolio company Trucksmarter is a load board platform designed for truck drivers. Besides offering an app for drivers to manage their business, Trucksmarter has also integrated a factoring product to enable drivers to get paid faster. This is a win-win: it’s better for truck drivers to get more value from one platform and consolidate their business management, and better for the financial institutions that are providing the factoring with more information on the risk.
However, we’re also seeing some inertia in the transition to embedded financial services as the low-hanging fruit has already been picked, so to speak. We’ve seen that the easiest financial products to embed, including payments and wallets / deposit accounts require very little individualized underwriting. For example, any ecommerce website can offer embedded digital consumer payments by leveraging Moov’s API (one of our portcos) within the checkout flow, and doesn’t have to individually underwrite each customer before paying digitally at checkout.
Contrast this to other categories that do require individualized underwriting, for example insurance, where we’ve seen the embedded applications by and large be more restricted to one-size-fits-all products like travel insurance and product warranties. Most individually-underwritten property and casualty (P&C) products, the lionshare of the P&C market, has eluded the embedded distribution model because we’ve seen that embedded distribution often requires a change to the way that the financial services product is manufactured as well. In the case of P&C insurance, it is usually too difficult to manufacture the insurance product (i.e. collect requisite data on the application, underwrite the applicant’s risk, present a quote, select and bind the policy) at a speed required for a delightful embedded customer experience. (Lots to unpack as to why this is, which is the subject of another blog post.)
The other reason we’re seeing some inertia is that incumbent financial services providers have not been sitting ducks; they’ve effectively been able to fight back by (1) matching the digital distribution methods of disruptors (largely enabled by other enabling fintechs), and (2) preserving superior economics through their scale, conservatism, and licensure/regulatory superiority.
What will it take to unlock the next level of innovation in financial services? Our experience with embedded financial services reveals that the solution must address the friction points in financial services manufacturing, and not just distribution. Generally, financial services products have three stages of the lifecycle:
How can we innovate on the ways that financial services are delivered? Here’s where I believe generative AI will come in: generative AI is the critical ingredient to make financial services products more accessible and more efficient. When we look at the anatomy of a financial services product, we can see the reality that the complexity in the process comes from the fact that it is a multi-party transaction with varying levels of trust and transparency requiring multiple data sources and multiple modes of interaction. Until recently, AI has by and large not been strong enough, robust enough, or flexible enough to be applied in this complex ecosystem. With generative AI, the innovation frontier has been stretched out and we’re seeing the calculus change.
The next frontier of “embedded fintech” will be embedding generative AI into financial services.
Let’s walk through 10 possible applications of generative AI within the manufacturing of a financial services product. This list is non-exhaustive, and highlights the broad scope of the opportunity. As a bonus, I asked Chat GPT to write one of these descriptions (can you identify which one?).
Distribution Process:
Manufacturing Process:
Servicing Process:
There are a few players that are well-positioned to take advantage of generative AI in the financial services world:
One possible critique of the list above of generative AI applications within financial services is that none of the examples above are revolutionary; rather, each is an opportunity to incrementally improve the process of providing financial services. This is a feature, not a bug! What we find so exciting in imagining the future applications of generative AI within fintech is that the layering of multiple of these incremental improvements can revolutionize the value we can capture from financial services products. Another way to think about it: if today’s generative AI can get to 90% accuracy and a human review can get to 100%, you are making a human 10X more productive and hitting the accuracy threshold required to deliver financial services.
A few pointers for early builders in this market:
We’re early in this journey and are excited to see this market unfold over the coming years. If you are building in this space or considering how to take advantage of generative AI within your fintech platform, we’d love to hear from you.
Special thanks to many who contributed to and pushed my thinking for this piece, including Annie Robertson Hockey, Animish Sivaramakrishnan, Erin Yang, Jill Greenberg Chase, Natalie Vais, Niko Bonatsos, Zach Smith, and my partners Christina Melas-Kyriazi, Dawit Heck, Matt Harris, Noah Breslow, Rak Garg, Sam Crowder, and Slater Stich.
Generative AI can make companies more efficient, but customers have more to gain from it than they do — including in banking, commerce and medicine.
The BCV fintech partnership shares our comprehensive perspective on how generative artificial intelligence is reshaping the financial services industry.
One aspect of the banking crisis is over, but generative AI stands to vanquish hundreds or thousands more small and mid-size banks.